Projects
Uncertainty is ubiquitous in all models and data. From stochastic modelling, where fluctuations play a central role in the dynamics of the process, to data collection, where measurement and sampling error permeate the data, it is central to understand the effects of uncertainty. The UQ project is centered on placing these elements in focus. We are a collaboration of nine Helmholtz institutes focused on a variety of topics.
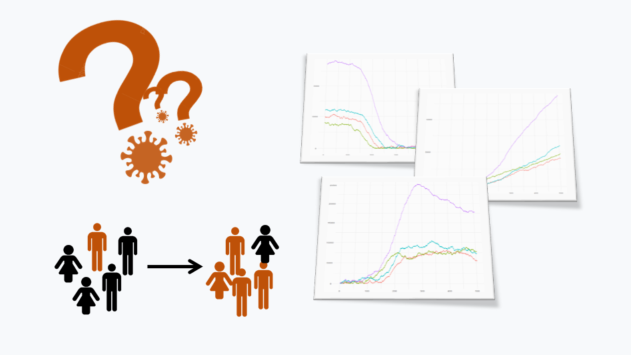
Tackling the numerous uncertainties regarding Covid-19
Researchers around the world aim to understand and fight the spread of the novel coronavirus, SARS-CoV-2. They face several uncertainties including unknown disease characteristics, underreported case numbers, false negative and false positive testing results, differing testing strategies and differing case definitions. Our aim in this project is to quantify these uncertainties arising from the stochastic models we use for the spread of the disease, by developing rigorous methods for that purpose. Image by: H. Yaqine
Seismic imaging with uncertain data errors
Seismic imaging is a process to produce an image of the Earth’s subsurface properties from seismic data. The geophysical inference of these images depends on the accuracy of the seismic data as well as its associated uncertainties. Thus, it is important to understand these uncertainties and how they influence the resulting properties of interest. In this study, we will develop and test novel techniques to quantify the uncertainties associated with seismic data. Image modified from Kufner et al. “Deep India meets deep Asia: Lithospheric indentation, delamination and break-off under Pamir and Hindu Kush (Central Asia).”
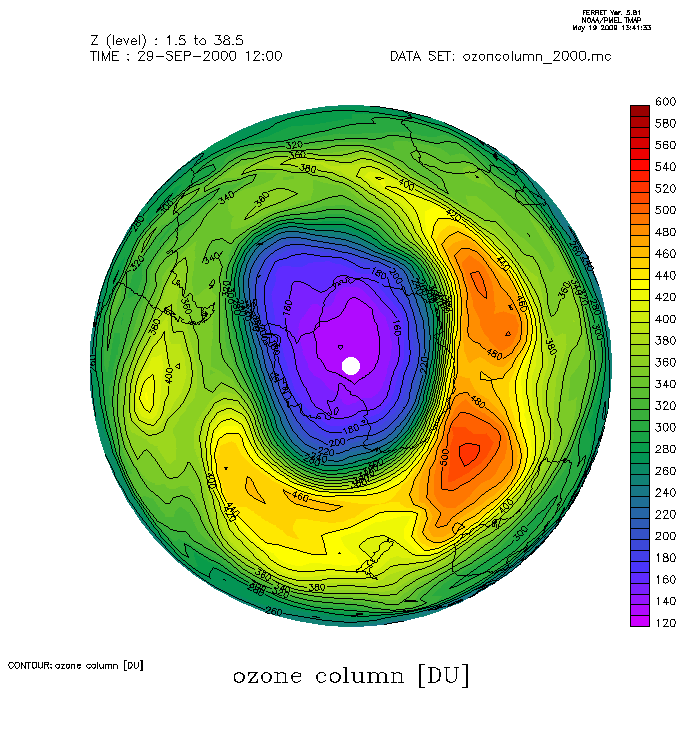
Quantifying uncertainties in projections of the Earth system
Uncertainty quantification plays an important role in better forecasting and understanding Earth system behavior. The focus of this project is to better predict ozone layer depletion in the atmosphere. The Montreal Protocol mandates the assessment of the ozone layer, which protects life on Earth from harmful ultraviolet (UV) radiation, and regulates the emissions of ozone-depleting substances. The current assessment is based on estimates of the expected return date of the (depleted) ozone layer to 1980-values, which are subject to uncertainties due to a methodology that relies on heavily smoothed and processed (model) data (identification). Here, we will explore novel methods of UQ to provide a new comprehensive assessment of alternatives for the UQ of return dates, which will be a policy-relevant contribution to the next assessment in the year 2022.
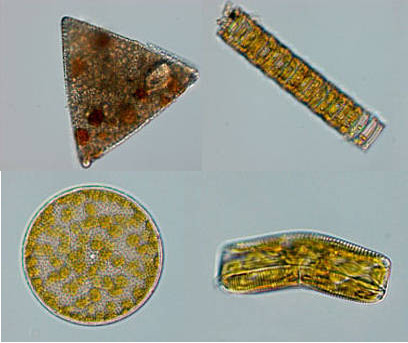
Ensemble data assimilation and uncertainty
Coupled ocean-biogeochemical models are built to better understand ocean ecosystems. REcoM-2 is a coupled ocean-biogeochemical model that has ~50 dynamic parameters with some uncertainties in their measurements. This project addresses the uncertainties in the REcoM-2 model using the technique of data assimilation. Data assimilation is a method of combining observations and data from numerical models to perform better analysis of underlying phenomena.
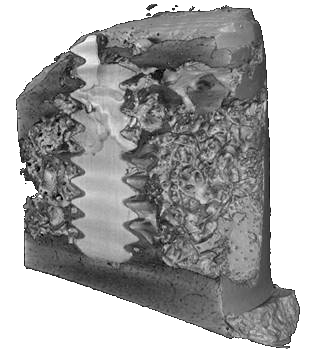
Uncertainties in models for Mg-based implant materials
Magnesium and its alloys are emerging as viable materials for temporary implant applications, e.g., in bone fracture healing. Computational models can be employed in order to predict implant degradation and the interaction of particular ionic components. In the current project, the UQ methodologies and principles will be applied to determine the uncertainties that appear in mathematical models simulating the in vitro degradation of biodegradable magnesium implants. Image by: B. Zeller-Plumhoff.
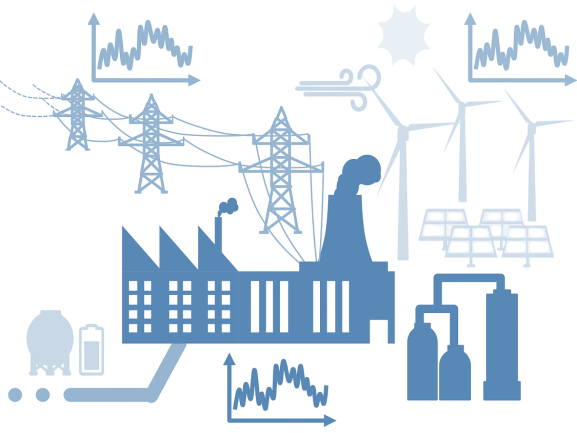
Operation and dynamics of energy systems under uncertainties
What are the consequences of a growing share of renewable energies in power-grid systems? How does the intermittent generation of renewable energy affect electricity markets? How can we apply this knowledge for the optimal operation of energy systems? Renewable energy sources are volatile in their energy output. This poses serious issues to both the operation and stability of power-grid systems. Furthermore, the volatile generation of renewable energy has a high impact on electricity markets as they react to imbalances of supply and demand by adapting their prices accordingly. It is therefore imperative to study the uncertainties associated with renewable energies to understand how they propagate through and alter power-grid systems. Additionally, the question arises of how to reach a cost-optimal schedule for the operation of energy systems under uncertain energy prices. Image by: FZJ-IEK-10
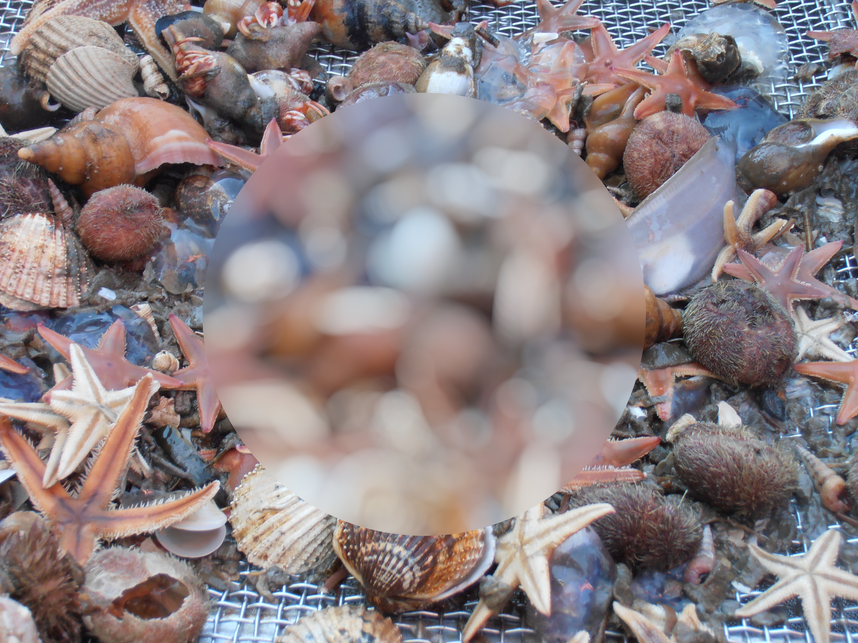
Propagation of uncertainty through ecological models
Within an ecosystem, how do changes in the physical environment affect the structure and function of its biological community? This question is becoming increasingly important as human activity accelerates environmental change across the planet. Ecological models that relate environmental variables to the structure of biological communities allow us to predict how these communities will be altered due to environmental change. Large amounts of biological and associated environmental data has been collected to this end. Unfortunately, there are numerous sources of uncertainty in these data. Misidentification of species, inconsistencies in sampling methodology, and error in the measurement of environmental variables are some of the issues that can affect long-term ecological datasets. Moreover, the choice of an ecological model is itself a source of uncertainty. We seek to understand how various sources of uncertainty affect our ability to make accurate predictions about community structure in the face of environmental change. Using long-term ecological datasets, we will choose the ecological model that best relates community structure to the measured environmental variables. This model will then be used to generate new datasets via simulation. Because these simulated datasets are generated from a known process (the model), we can then introduce various sources of uncertainty to see how they propagate through to the overall uncertainty of the response (predicted community structure). Image by: I. Kröncke.
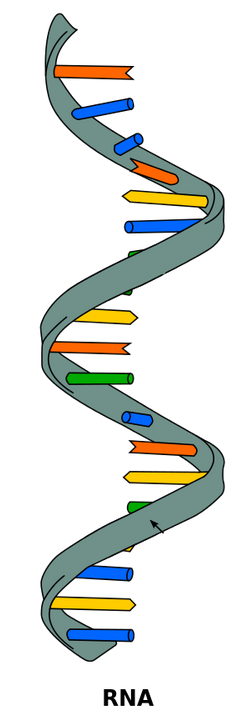
Uncertainty in RNA biology
Single-cell RNA sequencing data encounters uncertainty on both experimental and biological levels. For reliable analysis and biological interpretation of experimental results, it is crucial to identify and analyze uncertainty by appropriate probabilistic models. We derive distributions of sequencing counts from plausible mechanistic models and publicly available data and integrate them into relevant analysis methods. Image by: Sponk, CC BY-SA 3.0 , via Wikimedia Commons, adapted BY KW DNA deleted